Category: AI and ML
Automation and AI have changed how organizations conduct operations these days. As businesses continue to adopt automation within their operations, they are also using AI to enhance their strategies.
A study by Gartner Inc. reveals that 80% of business leaders believe AI-powered automation can be integrated into any business strategy.(1)
This power duo promises to boost productivity, minimize errors, automate manual tasks, accelerate decision-making via predictive analysis, enhance customer experiences and detect outdated patterns. These technologies collaboratively promote innovation and efficiency and help achieve unprecedented productivity within existing operations.
AI-powered automation is making a significant impact across various sectors, offering a wide range of benefits. Some key areas where AI is driving positive change:
Personalized Product Recommendations
AI algorithms analyze user behavior and preferences to offer tailored product recommendations. This not only enhances the customer experience but also boosts sales and engagement.
Anomaly Detection in Operations
AI can quickly identify unusual patterns or anomalies in large datasets. This is crucial for maintaining operational efficiency and addressing issues before they escalate.
Fraud Detection
AI’s ability to process vast amounts of data in real-time makes it a powerful tool for detecting fraudulent transactions. This not only saves companies money but also protects customers from financial harm.
Advances in AI
The latest generation of AI technologies is improving classification (sorting data into categories), estimation (predicting values), and clustering (grouping similar data points). This leads to more accurate insights and decision-making.
Added Value
As AI continues to evolve, it promises to deliver even more value to companies. Improved accuracy, efficiency, and scalability mean that businesses can leverage AI for a broader range of applications and achieve better results.
How Has AI Evolved Over The Years?
In recent years, AI has made significant leaps in natural language processing, machine learning, computer vision and robotics. Translating and giving out customized responses to human speech, image analysis, forecasting patterns based on operational data, and independently navigating environments are some capabilities of newer AI models. This evolution is due to AI’s advancements in data collection, processing, algorithms, and access to vast training data sets.
Hindrances AI and Automation Present Us With
Data and Algorithm bias, privacy constraints, lack of comprehensive data and cyber security risks related to automated decision-making are some hindrances AI and Automation present us with. Despite automating manual tasks, they raise numerous concerns around privacy, security, and the digital divide, especially during crucial processes like the development and deployment of credit processing systems or creating healthcare treatment plans.
The Indispensable Role of AI and Automation
Despite the hindrances, companies can leverage the powerful combination of AI and Automation in numerous ways-
Precision
One of the primary advantages of Automation and AI is their ability to ensure accuracy across all operations. These technologies can perform repetitive tasks at an accelerated speed. From data entry to quality control, they can help significantly in reducing errors and increasing overall output quality.
Enhanced Decision Making
AI goes beyond automation and provides valuable insights that can assist in making smarter decisions. By analyzing massive volumes of data in real time, AI can detect patterns, trends, and correlations that may not be noticeable to humans. It enables businesses to make informed decisions promptly, optimizing processes and allocating resources efficiently.
Scalability And Flexibility
As your organization expands and grows, your workload will also increase. Scaling up operations can involve extensive logistical tasks; this is where AI and Automation step in to make your job easy. These technologies offer unparalleled scalability, allowing you to handle increased workloads without proportionally adding to your workforce. Additionally, automation can be customized to fit specific business needs, ensuring adaptability in an ever-evolving market.
Reduced Human Efforts
AI and automation minimize human efforts by taking care of routine, manual tasks, enabling employees to focus on activities that require critical thinking, problem-solving, and innovation. This shift aids in the development of a more skilled and competitive workforce.
Uninterrupted Operations
Where human efforts end, AI and Automation take over. Businesses can maintain continuous operations around the clock, even across different time zones. These technologies benefit industries that require constant surveillance, like cybersecurity.
Customer Centric Approach
AI-powered chatbots and virtual assistants are changing the way businesses interact with customers. All these tools offer immediate responses to customer queries, enhancing the overall customer experience. AI analyzes customer data, helping organizations tailor their offerings to individual preferences, resulting in greater customer satisfaction and loyalty.
Competitive Advantage
Incorporating AI and automation technologies is not just about keeping up with the latest trends, it is also about gaining a competitive edge. By embracing these technologies, businesses can become leaders in their domain, offering faster, more efficient, and higher quality products or services.
To effectively implement AI and automation throughout an organization, it is essential to establish a well-defined strategy, allocate dedicated resources, and promote innovation and adaptability. Additionally, safeguarding data privacy and security should be of utmost priority, and employees may require training to acclimate to new technologies and processes.
The advantages AI and Automation bring to productivity and efficiency are undeniable. These technologies are no longer a luxury; they have become a prerequisite for businesses aiming to thrive in a rapidly evolving digital world. By harnessing the power of AI and Automation, you are not just streamlining operations – you are paving the way for a future where unprecedented productivity and efficiency reign.
So, why wait? Download our exclusive case study for best practices and implementation strategies. Let us help you embrace these technologies for accelerated business success.
References:
Generative AI is the new kid on the AI block that is scoring high marks in reducing the time taken in application development with improved productivity.
Generative AI is estimated to boost economic growth and value by $4.4 trillion, delivering powerful capabilities to non-technical users.
Despite the robustness of Generative AI models, they still present ethical challenges towards cybersecurity, balancing precariously between privacy and production.
The technology has both fascinated and alarmed security experts due to its potential to create realistic and sophisticated content.
This blog dives deep into the cybersecurity risks of Generative AI, what industry experts think of this emerging technology and some plausible solutions to prevent AI-driven cyber threats.
Cybersecurity Curve Balls
Generative AI has shown impressive capabilities in generating realistic results, synthesizing creative artworks, and even generating conversational responses indistinguishable from human speech.
However, these same abilities can be exploited for malicious purposes, such as creating sophisticated AI generated phishing attacks, spreading disinformation, or even fabricating information for altering public opinion.
Let us take a look at some curve balls Generative AI presents us with-
1. Data Privacy and Misuse
Generative AI systems use extensive data to determine and deliver accurate outputs. This substantial data collection and storage raises concerns about user privacy and potential misuse of confidential information.
2. Malicious Use Cases
Generative AI is vulnerable to AI-generated phishing attacks, providing scope for impersonation, or AI generated deepfakes, making it challenging for users to distinguish between real and fake information.
3. Bias And Discrimination
If the training data used for Generative AI models are biased, the generated content may reflect and amplify those biases, leading to discrimination or doctored outcomes.
4. Intellectual Property Concerns
With Generative AI capable of creating original content, there are concerns about intellectual property rights and copyright infringement.
5. AI-Augmented Cyberattacks
As AI evolves, AI-driven cyber threats could become more sophisticated, with attackers employing Generative AI to create ever-changing attack patterns that evade traditional security measures.
When asked about privacy concerns with Generative AI, Prashant Choudhary, Ey India’s Cybersecurity Partner, expounded, “Generative AI poses several privacy challenges. While some challenges have been discovered and many more are still coming out as more and more use cases pop up. And it is pervasive across all Generative AIs –Chat GPT, BERT, DALL-E, Midjourney, and so on.
The whole model is that you use training data, and then the AI comes out with whatever output it is supposed to give. It will give (output) based on the data that was used to train the model.
In this business, the data source is the internet and, there is a lot of web scraping involved, which brings the data to train these base models or the Large Language Models (LLMs).”( 1)
Plausible Preventive Measures
Although, we are unsure whether we can find foolproof solutions to mitigate Generative AI threats in cybersecurity, here are some plausible solutions we can adopt to address them.
1. Responsible Data Usage
- One preventive measure that can be taken is responsible collection, usage, and storage of data, while adhering to privacy regulations.
- Limiting data retention to the minimum for model training and actively seeking user consent can also be helpful.
2. Robust AI Verification
Developing AI-powered malware detection and prevention solutions for detecting and verifying the authenticity of the content delivered by Generative AI; can help user identify potential risks effectively.
3. Explainable AI
Be sure to implement techniques that make AI models transparent and open to interpretation. This allows users to understand the decision-making process and identify potential biases.
4. Collaborative Efforts
Encourage collaboration between AI and researchers, cybersecurity experts, regulatory authorities, and ethical governance bodies to determine and address ethical implications of Generative AI in cybersecurity.
5. Adaptive Cybersecurity Measures
Consistently update AI and cybersecurity policy to counter AI-driven cyber threats effectively. Also, use of AI technologies to develop proactive defence mechanisms against evolving threats can be beneficial.
6. Informed Consent
The use of Generative AI in various applications, such as virtual assistants, chatbots, or customer service interactions, raises questions about whether users should be explicitly informed when they are interacting with an AI system instead of a human.
In addition to these preventive measures, EY Cybersecurity partner, Prashant Choudhary believes “Synthetic data is a very interesting conversation to address all the copyright, legal, and other concerns when it comes to training LLMs. There are multiple interpretations of synthetic data, but for this conversation, I am assuming that synthetic data is basically when you generate a data using a computer and then you use that to train the LLM.”
He further explained that using computer-generated or synthetic data may appear to be a reasonable solution due to lower data costs, scalability, and the ability to generate multiple variants. However, this approach presents a challenge as the data will always reflect the algorithm used to generate it.
Industry experts are participating in discussions about using anonymized or tokenized versions of Personally Identifiable Information (PII) and other sensitive data.
With this approach, data is still extracted, but PII and other sensitive information are identified and replaced with anonymous labels to protect individual identities. This method can address privacy and other related issues and can be used to train LLM.
There are several regulatory authorities around the world who are giving their inputs around this issue. The NIST (National Institute of Standards and Technology) has developed the AI Risk Management Framework; the European Parliament is insisting on the EU Artificial Intelligence Act; the European Union Agency for Cybersecurity under discussion about cybersecurity for AI; the US Securities and Exchange Commission (SEC) are having conversations around AI, cybersecurity, and risk management.
Despite these factors, none of these solutions can create a solid defensive layer of protection against AI-driven cyber threats. These are not sure shot solutions but only preventive measures.
Generative AI presents an intriguing frontier in cybersecurity, offering both innovative solutions and ethical implications.
It is imperative to address ethical implications of Generative AI in cybersecurity, as the technology continues to evolve to guarantee a secure digital environment for all.
By encouraging a comprehensive discussion between stakeholders, implementing responsible AI and cybersecurity policies, and deploying innovative verification techniques, we can harness the power of Generative AI while mitigating its potential risks and fostering a safer digital environment for everyone.
For a deep learning of AI powered analytical tool development and implementation, please refer to our exclusive whitepaper resource on AI-based Gross To Net (GTN) Tool.
References:
- Cybersecurity in the age of Generative AI: solving the ethical dilemma. (EY.com).
Artificial Intelligence and Machine Learning have become revolutionizing strategies that businesses are incorporating into their operations. By leveraging data science consulting services, organizations are striving to tread ahead of their competitors.
In this blog, we will discuss how processes like document processing, market predictions, and CRM (Customer Relationship Management) services can be accelerated using AI and ML tools. We will also discuss bottlenecks and hurdles like biased algorithms and the need for extensive data preparation, which organizations must overcome when using AI-powered tools.
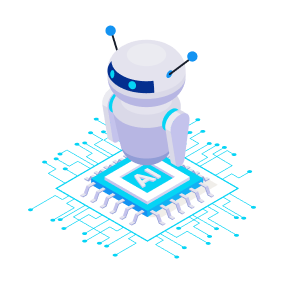
Let us look at some statistical information that has been made available in recent years on data science implementation:
- A survey by Gartner Inc.(1) recorded that 37% of companies have implemented AI in their operations. The adoption rate of the technology has tripled in the last year.
- Investment in AI by leading businesses continues to skyrocket by 91.5%.
- Gartner Inc.(2) predicts that by 2027, Chatbots and Virtual Customer Assistants will become the primary customer service channel for businesses.
- Deloitte(3) reports that 82% of companies that have adopted AI and cognitive technologies early on have noticed a substantial increase in ROI from their production-level projects.
- Accenture(4) reports state that AI can increase profitability by an average of 38% across 16 industries by 2035.
- A report by McKinsey and Company(5) a data analytics company, states that there has been an increase in AI adoption rate by 25% in businesses that operate in the healthcare, pharmaceuticals, finance, and retail sectors.
- According to a PWC study(6), 72% of business leaders believe that AI will be advantageous for their enterprises in the future, with 67% believing that AI and data science consulting services will open new doors of opportunities for them.
AI Strategy Components For Businesses:
In addition to tools and expertise, organizations must strategize how to incorporate AI and ML tools to derive maximum benefits. In this blog, we will look at three key strategy components that businesses must consider:
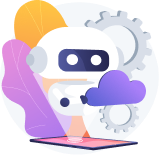
Business Case:
Before investing in AI and ML technology, organisations must establish a case study to convince stakeholders of an AI investment. Businesses must research case studies or hire data analytics companies concerning AI adoption and how their operations can benefit by using these technologies.
Leadership Support:
For utilizing organizational resources, leadership support is crucial. Leaders must communicate with teams, emphasizing the need for AI in their operations and how an effective strategy can contribute to the overall growth of their organization.
Change Management:
All organisational teams, departments, and leaders must collaborate and be willing to expend time and effort for the success of an AI strategy. Teams and departments must prioritize tasks and brainstorm ideas for an effective implementation process.
The Starbucks company is one such real-world examples of a business that has successfully utilised Machine Learning algorithms in operations such as digital marketing, procurement processes for improving sales and other functions of the business.
Hurdles businesses must overcome while utilizing Artificial Intelligence and Machine Learning
Although AI and ML can make your day-to-day business operations easy to manage and conduct, utilizing these technologies come with their own set of challenges.
- One of the biggest hurdles that businesses must overcome, is the need for extensive data preparation. For these technologies to operate effectively, businesses are required to have clean, relevant, and diverse datasets to train these algorithms to analyze the data. It can be a time-consuming and expensive process for businesses that have substantial and complex datasets.
- These technologies also pose a threat of presenting biased algorithms. For algorithms to deliver unbiased results, businesses must ensure that their data sets accurately represent their target audience and are unbiased. This is particularly crucial for finance and healthcare sectors as mismatched data can result in grave consequences for individuals and society.
Strategies such as investing in data quality and governance, using diverse datasets to train algorithms and training employees for new roles can be beneficial in addressing these challenges. Using low code platforms such as Microsoft Power Platforms that provide SaaS offerings can make the journey of adopting AI technology seamless for businesses.
Tools that businesses can use for implementing Artificial Intelligence and Machine Learning

- Tensor Flow: Tensor Flow is an open-source software library developed by Google for building and training machine learning models. It is extensively used by developers and researchers for several applications from image recognition to natural language processing.
- Amazon Web Services (AWS): AWS provides businesses with various AI and ML tools such as Amazon SageMaker for building ML models, Amazon Recognition for image and video analysis and Amazon Comprehend for natural language processing.
- Microsoft Azure: Microsoft Azures comes with an extensive set of AI and ML tools such as Azure Machine Learning for building ML models, Azure Cognitive Services for natural language processing and Azure Data bricks for data engineering and ML.
- Google Cloud AI Platform: Google Cloud AI Platform comes with a range of tools and services that help in building and deploying ML models that include Tensor Flow, Keras and AutoML.
While undertaking the process of selecting an AI and ML tool or platform, business must consider whether these tools are specific to their needs and requirements and if they have the proper expertise to effectively implement these technologies.
Businesses must also ascertain the level of complication a problem presents. If it is a simple text analysis, a basic data analysis or an image analysis, businesses can opt for low code platforms like Microsoft Power Platform or other intelligent process automation platforms like UIPath or H2O.
What’s next for Artificial Intelligence and Machine Learning in Business .
The evolution of AI and ML technologies provides businesses with new avenues to explore when it comes to their usage in their operations. AI and ML can be used for supply chain optimization and automation, for improving fraud detection and enabling more personalized and targeted marketing campaigns.
Businesses are required to be cautious and mindful of the ethical and social implications that these technologies present. Especially in the areas of privacy, bias, and job displacement. Businesses need to consider investment in transparency, accountability, reskilling and upskilling their work force for newer roles while adopting data science technologies.
The technologies of AI and ML have the potential to transform how businesses will operate and compete in this digital age. By effective utilization of these technologies, businesses can stay ahead of the curve in their respective industries.
For more understanding about AI and ML strategies, check out our informational video on “Top 3 AI ideas you can use for your business.”
Business process automation now becomes the new norm at the workplace to cope with a fast-paced economy and growing challenges for modern business. While a few organizations may very well consider automating their IT tasks, RPA likewise means to help them rehashing the way they work together, uplifting their consumer loyalty and fortifying employee work values. The necessity of bringing automation has given a boost to Robotic Process Automation (RPA) solutions. But AI (Artificial Intelligence) comes into the center stage as entrepreneurs craving more assistance from robotic process automation. Near future, it would be hard to imagine the RPA solution without AI integration.
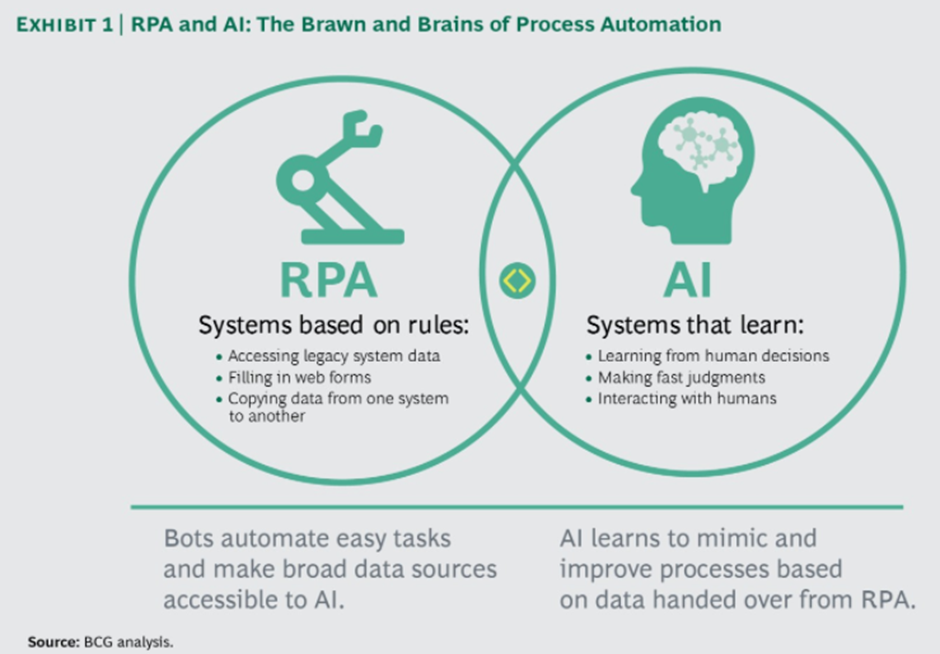
According to Forester’s 2019 predictions, the combination of RPA and AI is becoming the new strategic
investment in the corporate world. AI and RPA are emerging automation technologies with enormous potential. The combination helps businesses in achieving tremendous growth in such a competitive market.
You may curious why a business should combine these two technologies?
The reason is simple- RPA has no capability of cognitive awareness, and AI brings it in the customized solution. This combination of AI and RPA adds up to intelligent process automation (IPA). In interest to machine learning algorithms and RPA, IPA also incorporates process management software, natural language processing, creation, and cognitive agents, or “bots.” According to McKinsey, IPA can –
- Add up to20 to 30% improvement in proficiency,
- Reduce the processing time by 50 to 60 percent!
Make an improvement in ROI in triple-digit percentage. TMR (Transparency Market Research) has predicted RPA will be prevalent in the corporate world that its market to reach up to $16 billion by 2024. On the other hand, AI focused on imparting intelligence in the machines and connected devices through speech recognition, decision making, and predictive analysis.
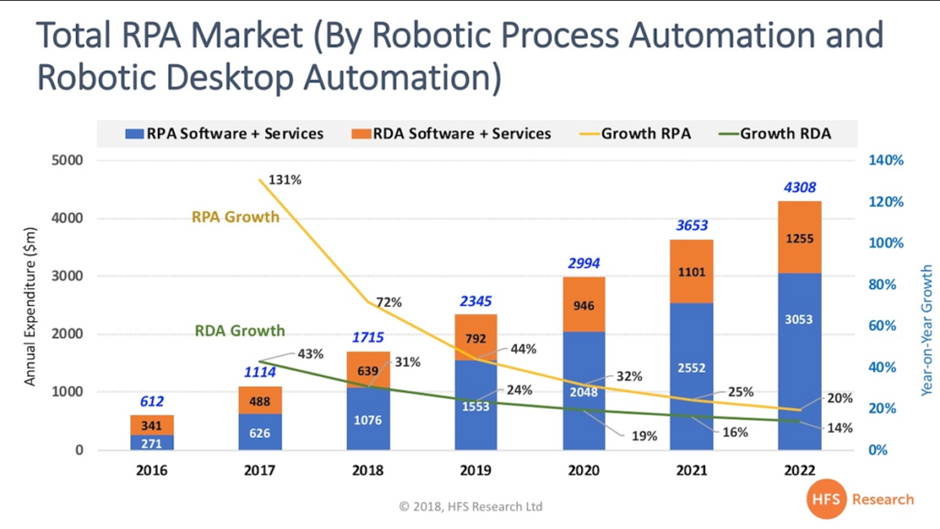
As AI is gaining ground swiftly across the world, it can be integrated with other emerging technologies like IoT and Blockchain to realize the futuristic concepts of smart cities and secure transactions sequentially. McKinsey foresees AI to deliver around $3.5 to $5.8 trillion in value to the world per annum.
How AI enhances RPA services
AI and RPA combination enables modern enterprises to leverage the benefits of both these technologies in accomplishing incomparable tasks with higher efficiency. It is like something beyond the range of a customized RPA solution because it develops to perform the same and repetitive type of work.
At the point of a substantial change is anything but a reasonable alternative for companies, RPA can go about as a facilitator to enable organizations to grow and include value. It also permits them to appear with strategic frameworks around investment they’ve efficiently made into their custom frameworks. The assimilation into AI is the following stage of this granular, more responsive type of change, with more business exercises either completely or partially automated by progressively refined means. It is known as cognitive RPA or CRPA.
RPA alone imitates human action through different AI capabilities, machine vision, speech recognition, and pattern identification capacities and can deal with organized, semi-organized, and unstructured data. However, infused with machine learning gives robots a chance to figure out how to process and enhance tasks that ensure probabilistic conduct.
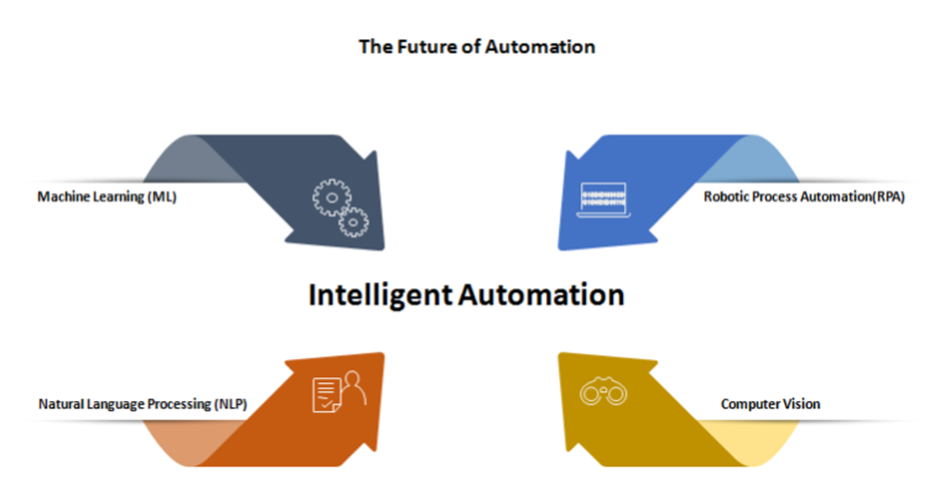
AI integrated Robotic Process Automation solutions bring the capability of gathering and sharing valuable information with different systems for better decisions. Customized RPA solution can utilize data fetched by AI and performs the complex task with ease. Adding up, AI, commonly with cognitive technologies and ML (Machine Learning), enables robotic process automation by preceding a human response in the workflow.
The humble bot
Organizations aren’t looking for just a bot. They have been looking for a platform with orchestration and integration where, they could readily adopt modern technologies and combine them with intelligence. Now, most organizations are strategizing to automate most of the steps of a business process and then applying a different level of intelligence. Hence, when picking vendors for their bots, the enterprises keep their eye towards that future.
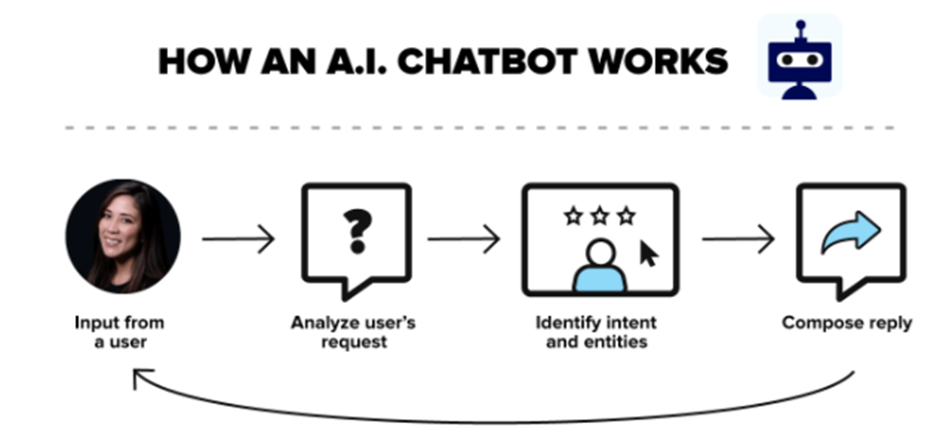
Just like, Germany’s ZF Group, an automotive supplier has started applying intelligence to its business processes just over a year ago and created a bot to answer the most repetitive questions. According to the company’s IT manager around their corporate communication, they have a lot of repetitive work. Furthermore, they receive a lot of emails with repetitive questions coming into their inboxes. Automated integrations and orchestration were their first goal, and they also wanted a platform with built-in checks and balances. Finally, they choose a bot platform that offers a management, governance, and language support layer underneath the bots, called stateful network for AI process (SNAP), which will stop a bot if it demonstrates anomalous behavior.
Real-time Decision support system
Decision points are another way to add intelligent decision points into a traditionally automated business process. Nevertheless, cognitive technologies and machine learning direct out to be even more severe. In general, when AI technologies are integrated with RPA stages will automate the emotional and judgment-based process.
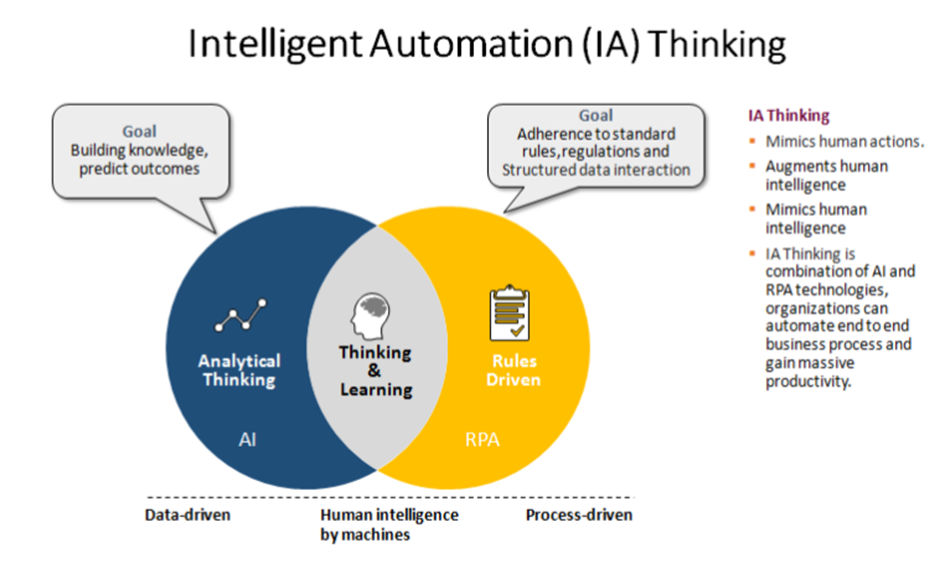
Just like American Fidelity Assurance, an insurance policy provider turned to UiPath, an enterprise RPA vendor, and AI platform Data Robot, to add intelligence to its processes. A system that can manage and execute real-time decisions through AI is the best option for automating complex decision processes. Applying high-level analytics and machine learning those systems to become intelligent and continually adjust and discover from new, contextual data. They set up a real-time decision-making system in form of RPA that helps to transfer highly complex problems in real-time applying AI-based analytical decision-making.
For investment firms, real-time decision-making can analyze thousands of business inputs, constraints, and options and arbitrate between multiple internal targets and trading decisions. It can apply an analytical constraint-based optimization approach to models across thousands of possible actions – whether it be specific content, an offer, a price, a sort order, or a recommendation – and determine the best outcomes for the organization. sometimes it qualifies those choices upon certain criteria from profitability, risk, or revenue perspective.
Sometimes, the traditional approaches to RPA may hit a decision point that is too complex for simple automation. Considering the facts, organizations are also looking at using AI for process mining to automate process discovery rather than have business analysts figure out what happens in the company.
Process mining
RPA-Robotic Process Automation is transforming the system many organizations function. Process Mining enables companies to holistically experience their methods and recognize process advancement possibilities to boost efficiency and overcome costs. Its 360° view of processes enables hyper-automation—standardizing and accelerating enterprise automation using technologies, such as machine learning (ML) and artificial intelligence (AI).
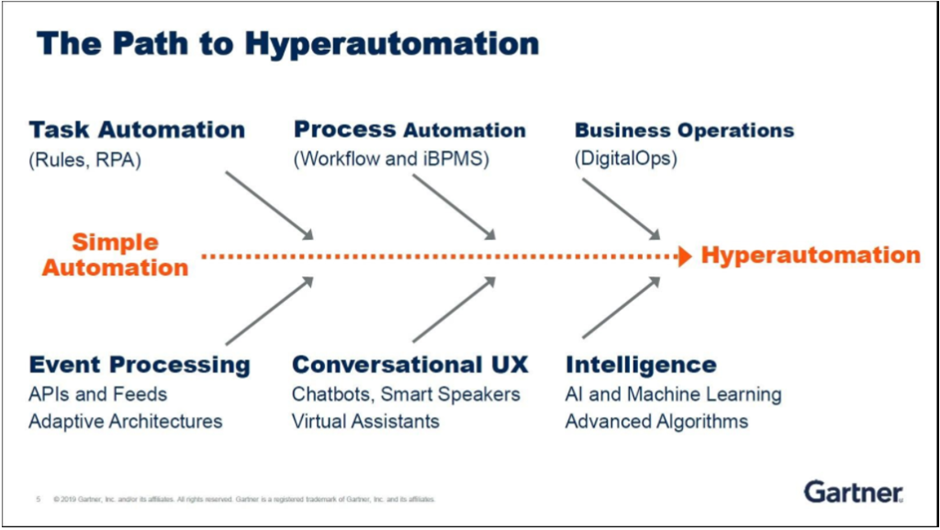
The conventional way to business process management involves business analysts talking to managers and employees, carrying audits, then creating charts that illustrate the organization’s various business processes.
Sumeet Vij, director of the strategic innovation group at Booz Allen Hamilton, has mentioned how clients are presenting process workflow automation and how things happen, bottlenecks are different. When it comes to process mining, machine learning helps people experience a picture of how things are actually happening. New tools will update the processes and spot anomalous behavior in real-time with the evolvement of business.
Sometimes, a business process may be viewed as charts, such as Visio diagrams, and managers can drill down into the process, down to the level of individual transactions.
Business process analytics
Some organizations have sufficient business process data that they can instantly view the overall picture of what’s happening and make analyses and forecasts. Organizations are taking the exhaust from the RPA process and trying to capture that, learn from it, and make those processes smarter.
Seann Gardiner, senior VP of business development at Data-Robot (an AI platform provider), mentions if a company has a strong focus on process-level automation and can manage that data, then it might be ready. But strong leadership is also mandatory to make the process successful. Business leaders who believe in automation and an AI-first mentality can make the organizational changes needed.
The use-cases of intelligent automation will likewise keep developing as new AI procedures and solutions enter the marketplace, drastically changing the future working environment. It can perform even complex and unique business actions like humans as an advanced solution. However, there is no point in believing that this combination will replace humans as they remain the controller of the RPA +AI combination by building the rules and manage the operation. The combination of RPA and AI had aimed to bring a digital transformation in the companies and adding more value over the period.
For organizations to use this innovation completely, they initially need to see how these solutions can change their procedures and apply strict governance to settle on beyond any doubt their decision-making is paramount. If organizations attempt to actualize these connected yet granular solutions over their companies, it will enable them to drive a double speed change and pursue new heights in Business and IT maturity level.